A Comprehensive Guide on Conformed Dimensions
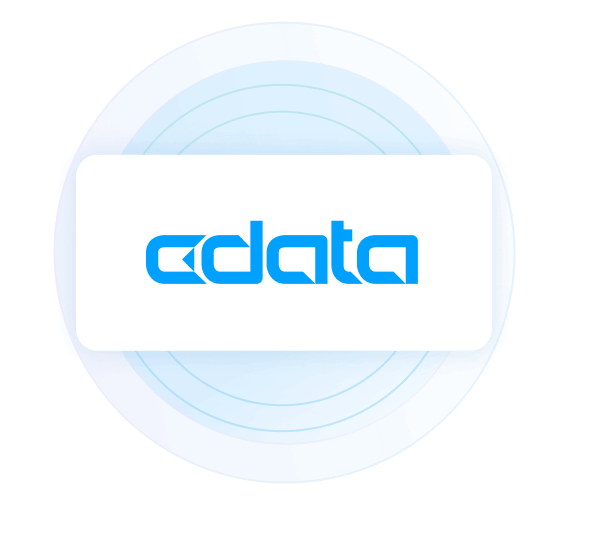
Businesses rely heavily on insights drawn from vast amounts of information. To make data analysis efficient and accurate, organizations need to establish a unified framework that ensures consistency across multiple datasets and sources. One key concept that helps achieve this is the conformed dimension. Conformed dimensions serve as the backbone of many data warehousing and business intelligence (BI) systems, allowing different datasets to interact seamlessly, enhancing both the reliability and coherence of analysis.
What is a conformed dimension?
A conformed dimension is a specific type of dimension used in data warehousing that is consistent across different fact tables or subject areas. This consistency ensures that data can be accurately compared, aggregated, and analyzed across various data sources. For instance, if a company has multiple datasets representing sales, customer support, and inventory, a conformed dimension, such as "Time" or "Product," ensures that these datasets reference shared definitions. This way, regardless of the dataset, the "Product" or "Time" dimension will always mean the same thing, facilitating cross-functional analysis.
A conformed dimension acts as a common reference point that aligns data across different systems. This alignment enables consistent reporting, which is vital for business intelligence, as it removes ambiguity and ensures all stakeholders are working from the same understanding.
5 advantages of conformed dimensions
The adoption of conformed dimensions offers a range of benefits that strengthen data integrity and the efficiency of data analysis. Here are a few of the primary advantages.
Consistent data
Conformed dimensions bring consistency to data interpretation across an organization. When different departments or datasets use the same definitions for key data points—such as "Customer," "Time," or "Region"—data analysts and business users can trust that they are making decisions based on unified and accurate information. This consistency is crucial for any organization that values reliability in its reporting.
Reduced data duplication
Establishing a conformed dimension allows organizations to avoid duplicating dimensions in different datasets, saving storage and ensuring that only one version of each key data attribute is used. This also simplifies data management, as any changes to a dimension only need to be applied once, without the risk of creating inconsistencies across systems.
Efficient analysis
With conformed dimensions, organizations can perform faster and more accurate analyses across multiple data sources. Since dimensions are standardized, cross-functional reporting and comparisons are more straightforward because there is no need for additional processing to reconcile data differences. This standardization reduces the burden on both data analysts and IT teams, enabling quicker insights and faster decision-making.
Easier integration
Conformed dimensions simplify the integration of data from different sources. With standardized definitions, combining datasets—whether during data integration projects or ETL processes—becomes a more streamlined task. Data from different sources can be related through shared dimensions, making integration a less complex and error-prone process.
Enhanced data quality
Conformed dimensions ensure high data quality by reducing the possibility of conflicting definitions. When each department works with identical definitions and data attributes, errors due to interpretation differences decrease. This consistency makes enforcing rules and standards easier across conformed dimensions, enhancing data governance.
5 disadvantages of conformed dimensions
While conformed dimensions offer numerous benefits, they also present certain challenges. Understanding these challenges can help organizations effectively prepare for and manage the complexities of implementing conformed dimensions.
Managing changes and updates
One of the significant challenges with conformed dimensions is managing changes and updates. When a dimension needs to be updated or modified, it can impact multiple datasets and processes. For instance, if the attributes of a "Customer" dimension are updated, ensuring all associated datasets reflect these changes is critical. This level of maintenance requires rigorous change management practices to prevent discrepancies and maintain data consistency.
Cross-functional collaboration
Implementing conformed dimensions requires strong collaboration between various departments or functions within an organization. Since dimensions like "Customer" or "Product" may be interpreted differently across teams, achieving consensus on standardized definitions can be challenging. This need for cross-functional alignment often requires buy-in from leadership and effective communication across departments.
Resource requirements
Developing, implementing, and maintaining conformed dimensions demands a significant investment in time, talent, and technology. Organizations need to invest in skilled professionals, robust data management tools, and infrastructure to ensure conformed dimensions function as intended. For smaller organizations with limited resources, this investment may be difficult to justify.
Potential for rigidity
Conformed dimensions can introduce some rigidity in data structures, which may not always be ideal for rapidly changing business environments. As organizations evolve, so do the ways they use and interpret data, and conformed dimensions may occasionally require revisions to remain relevant. This can slow down the response to certain changes, especially when agility is required.
Complexity in governance
Effective governance is essential for the successful implementation of conformed dimensions, but this can add layers of complexity to data management processes. Organizations must establish clear ownership of dimensions, create and enforce policies for data usage, and monitor compliance. These governance practices can be demanding, especially in large or fast-growing organizations.
How to create conformed dimensions in 5 steps
The process of building conformed dimensions can be broken down into several key steps that provide a foundation for consistent, high-quality data analysis.
-
Identify common dimensions
The first step in creating conformed dimensions is to identify the dimensions that are common across multiple datasets or business areas. Examples of common dimensions include "Time," "Product," "Customer," and "Location." Determining which dimensions should be conformed ensures that the focus is on those that will provide the most value to the organization.
-
Set attributes and hierarchies
After identifying common dimensions, define the attributes and hierarchies associated with each dimension. For example, a "Customer" dimension may include attributes like "Customer ID," "Name," and "Region." Similarly, a "Product" dimension may contain hierarchies like "Category," "Sub-Category," and "SKU." Establishing these attributes and hierarchies helps ensure that each dimension captures the required details for accurate reporting.
-
Establish standards and definitions
To ensure conformed dimensions are used consistently, organizations should establish standards and definitions for each dimension. Documenting these standards creates a single source of truth that all departments can refer to. This documentation should include details about each attribute, acceptable values, and any transformations or validations applied to the dimension.
-
Create a master data source
Once dimensions are defined and standardized, create a master data source or repository that serves as the authoritative source for each conformed dimension. This master source should be maintained and managed carefully, with strict version control to handle updates. Having a single repository helps simplify the integration of conformed dimensions across various systems.
-
Implement conformed dimensions
With definitions and standards in place, implement the conformed dimensions across relevant datasets, tables, and data sources. This often involves modifying ETL processes to integrate the conformed dimensions and ensure they are applied consistently. Testing is essential at this stage to confirm that the conformed dimensions are functioning correctly and meeting reporting needs.
3 conformed dimension examples
Below are a few examples of commonly used conformed dimensions and how they might be applied in data analysis.
- Time dimension: This dimension is crucial for tracking events, transactions, and performance over time. It includes attributes such as "Year," "Quarter," "Month," "Week," and "Day." By conjoining datasets with the same time dimension, organizations can perform longitudinal analyses and observe trends over specific periods.
- Customer dimension: A customer dimension contains details such as "Customer ID," "Name," "Location," and "Customer Type." This dimension allows organizations to analyze customer behavior, segment customers, and track customer engagement across different datasets, such as sales and customer service.
- Product dimension: Common in retail and manufacturing, the product dimension includes attributes like "Product ID," "Category," "Brand," and "Price." With a conformed product dimension, businesses can analyze sales, inventory, and supply chain data in a unified way, providing insights into product performance.
CData Virtuality
CData Virtuality offers a powerful solution for organizations looking to create, manage, and maintain conformed dimensions with ease. By providing a unified platform for data integration, virtualization, and data management, CData Virtuality simplifies the process of implementing conformed dimensions, reducing the complexity of data analysis.
Explore CData Virtuality
Take a free, interactive tour of CData Virtuality to see how you can streamline your data architecture and improve data consistency for accurate, efficient reporting.
Tour the product