Data Quality: Learn All About its Key Dimensions, Why It Is Important & How It Differs from Data Integrity
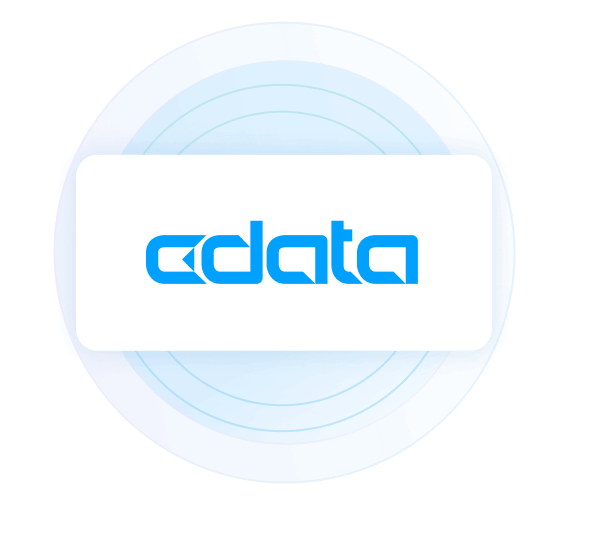
In today's data-driven world, you can’t overstate the importance of data quality. As organizations increasingly rely on data to drive decision-making processes, ensure customer satisfaction, and gain a competitive edge, the need for high-quality and reliable data has become paramount. Bad data leads to a myriad of issues, including faulty analysis, misguided strategies, and potential financial losses. As data volumes grow from a multitude of sources, maintaining data quality has become a significant challenge. This means that investing in robust data quality management practices has become essential so organizations can harness the full potential of their data assets and drive business success.
In this article we define data quality and explore its key elements. We’ll touch on why it’s so important in today’s business climate, and clearly explain the difference between data quality and data integrity.
What is data quality?
Data quality is the degree to which data meets its users’ expectations on accuracy, completeness, consistency, timeliness, relevance, and accessibility. It encompasses a variety of aspects, including the absence of errors or inconsistencies, the accuracy of values, how appropriate the data is for its intended use, and the ease of data access and interpretation. Data quality ensures that information is trustworthy and fit for purpose, which means users can analyze it and make decisions with confidence.
Data quality is critical across many business functions, including marketing, finance, and operations, because it directly impacts decision-making processes and organizational performance. When they know that their data is reliable and fit for purpose, businesses can drive innovation, improve performance, and maintain a competitive edge.
In marketing, good data is essential for understanding customer preferences, behaviors, and market trends. Bad data in marketing efforts can lead to wasted resources, ineffective targeting, and missed engagement opportunities.
In finance, data quality is crucial for financial reporting, forecasting, and risk management. Reliable data ensures compliance with regulations, facilitates accurate forecasting and budgeting, and contributes to strategic decision-making. Inaccurate or inconsistent financial data can lead to compliance issues, financial mismanagement, and decreased stakeholder confidence.
In operations, reliable data lets organizations monitor performance metrics, identify areas for improvement, and make informed decisions that streamline operations and enhance productivity. Poor data quality in operations can result in inefficiencies, production delays, and compromised product quality, which can significantly affect customer satisfaction and profitability.
Let’s take a quick look at a real-world example: Retail companies rely on sales data to make inventory management decisions. If a company's sales data contains errors, such as duplicate entries, missing values, or inaccurate product descriptions, the data inaccurately reflects sales trends and customer preferences. The company might use this data to mistakenly identify certain products as top sellers and others as slow-moving, which could lead to incorrect inventory stocking decisions and directly impact the company’s bottom line.
What are the 9 dimensions of data quality?
The term data quality dimension describes the measure of the quality of data being analyzed. Here's a list of nine core data quality dimensions:
- Accessibility: The ease with which authorized users can access, retrieve, and use data. This ensures that data is available and readily accessible when needed.
- Accuracy: The correctness and precision of data. It ensures that values are error-free and correctly represent the real-world objects or events they describe.
- Completeness: The extent to which all required elements are present in a dataset (without missing or null values), so that the dataset contains all necessary information.
- Consistency: The extent to which data is free from discrepancies or contradictions within and across different datasets or data sources, thereby maintaining uniformity and coherence.
- Precision: The level of granularity or detail present in data values. This ensures that the data is detailed and specific enough for its intended purpose.
- Relevance: The extent to which data is applicable for the intended purpose or use case. This ensures that only pertinent information is included in analysis or decision-making processes.
- Timeliness: The relevance of data in relation to the specific timeframe or context in which it is needed, so that the required data is up-to-date and suitable for analysis.
- Trustworthiness: The confidence and reliability associated with data, considering factors such as data source, quality assurance processes, and potential biases or errors. This ensures that data can be relied upon for decision-making and analysis.
- Validity: The degree to which data conforms to predefined rules, standards, or constraints. This ensures its accuracy and reliability.
Why is data quality important?
Data quality ensures the reliability and usefulness of data for organizational decision-making, operations, compliance, and innovation. Decisions made based on high quality data lead to better strategic planning, improved operational efficiency, and increased competitiveness.
With good data, organizations better understand their customers, anticipate their needs, and deliver more personalized experiences. Better understanding leads to higher customer satisfaction and loyalty. Businesses can also identify and mitigate risks effectively, across the organization. In addition, organizations that consistently deliver accurate and reliable information gain trust and credibility with all their stakeholders, which enhances their reputation and brand value.
Poor data quality can lead to inefficiencies, errors, and rework, which result in wasted time and resources. Bad data can also damage reputations and hurt profitability. High-quality data ensures that insights derived from data analysis are trustworthy and actionable, so organizations can make informed decisions, identify opportunities and, ultimately, increase profits.
How does data quality differ from data integrity?
Data quality and data integrity are closely related concepts, but they have distinct focuses within the broader scope of the data’s "fitness for use."
As you’ve seen, data quality refers to the overall condition or state of data, encompassing dimensions such as accuracy, timeliness, relevance, and accessibility. It assesses how suitable the data is for its intended purpose or use by considering factors like correctness, reliability, and relevance to the task at hand. It also involves evaluating and improving the quality of data across multiple dimensions to ensure that it meets the needs and expectations of its users.
Data integrity specifically refers to the accuracy, consistency, and reliability of data throughout its lifecycle. It ensures that data remains consistent over time, regardless of how it’s stored, transferred, or manipulated. It also ensures that data is free from errors, corruption, or unauthorized alterations. It’s primarily concerned with preserving the reliability and trustworthiness of data, guarding against data loss, corruption, or unauthorized access, and ensuring that data remains accurate and consistent for its intended purpose.
The bottom line is that both concepts are essential for maintaining the overall quality and trustworthiness of data within organizations.
Data auditing for accountability with CData Connect Cloud
CData Connect Cloud provides centralized access to data so you can streamline your monitoring and auditing processes to ensure data transparency, accountability, and quality across your organization. With CData Connect Cloud, you can integrate disparate data sources into a single, unified platform, which provides a comprehensive view of your organization's data landscape. Take control of your data ecosystem today with CData Connect Cloud and unlock the full potential of your data-driven initiatives!
Try CData Connect Cloud
Try Connect Cloud for free today to learn how to uplevel your data strategy.
Get a trial